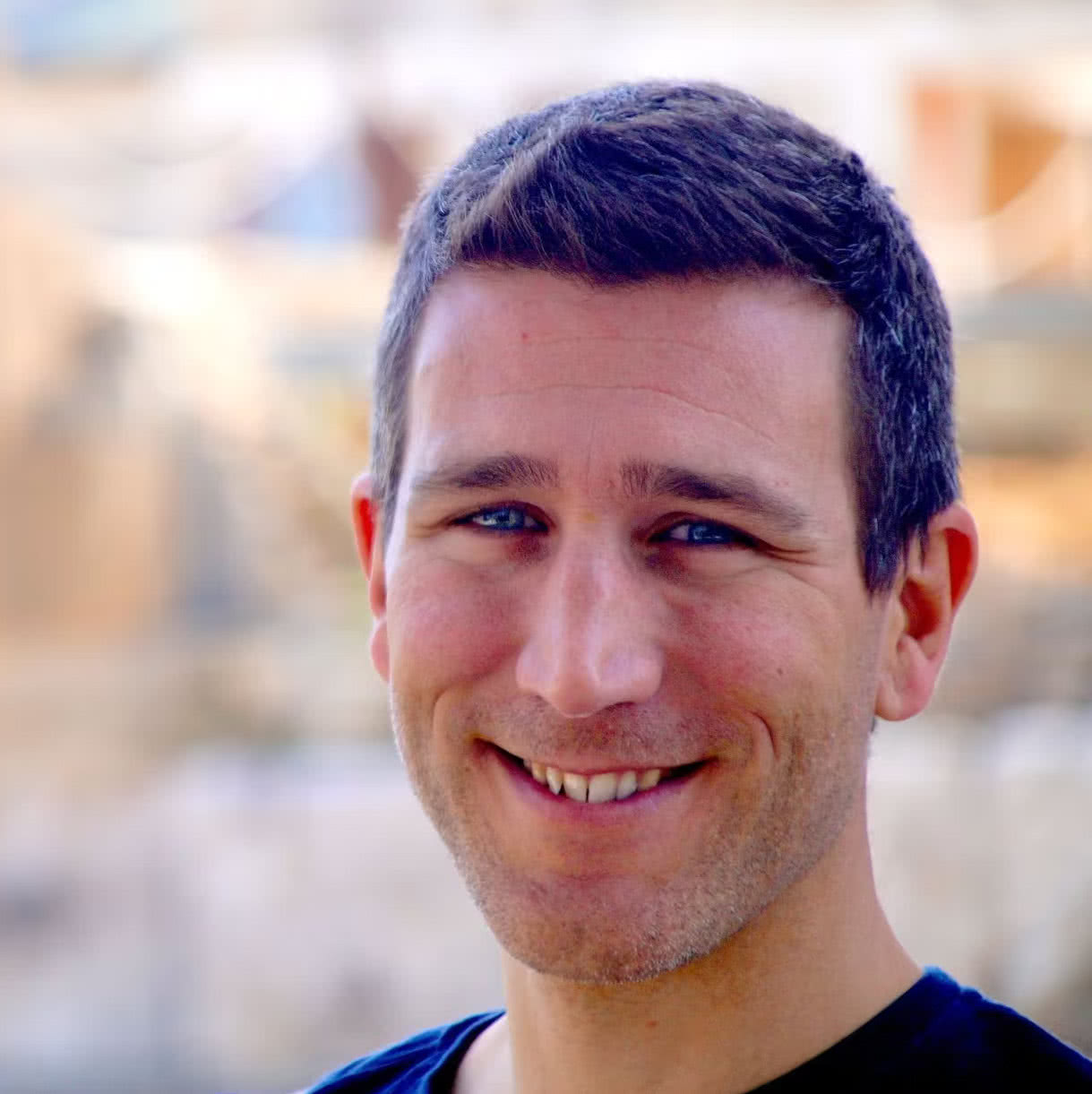
Álvaro’s career journey spans across the fields of theoretical physics, computational neuroscience, and machine learning. Initially focusing on the interference of entangled material particles, and later on models of memory sequences through theoretical neural-network models and data analysis, his work has been deeply interdisciplinary. His experience includes a stint at the German Aerospace Agency and the European Southern Observatory. During a postdoc in Oxford, he developed an interest in machine learning, applying Bayesian time-series models to classical signal analysis techniques. Álvaro’s professional path has oscillated between academia and industry, exploring the balance between theory and practical application across various scientific fields. His recent work involves applying machine learning to mechanistic models for solving inverse problems through simulation-based inference methods.
At the ml ⇌ science colab, Álvaro and his team leverage machine learning methods for scientific discovery in fields as diverse as archaeology, paleo-climatology and high-energy physics.